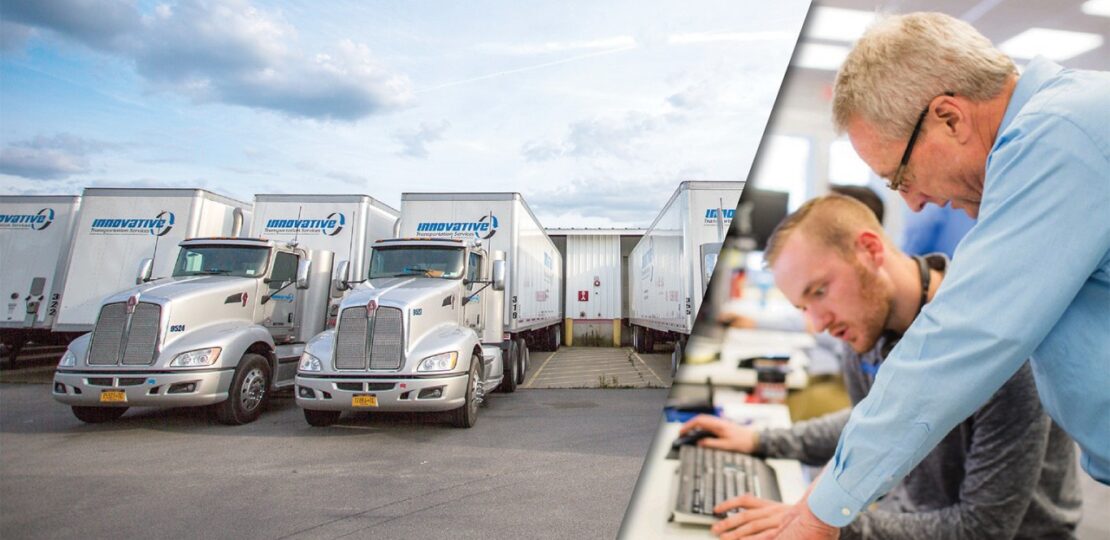
Machine learning and Artificial Intelligence have been the keywords for the last 10 years, there are newer buzzwords being added almost every month relating to ML and AI. But as a Data Analyst in a logistics brokerage firm called Sonwil Logistics, I have been working with logistics data and figure out ways to implement ML in logistics. There are some key areas where ML can help any logistics company. And while there can be many use cases, these are the major areas which will have an impact on the bottom-line of a company.
A) Route Optimization: Most carrier companies rely on past experiences, information through their network, gut feeling to plan their tractors and trailers for the day/week/month. While big names like JB Hunt, CHR and XPO etc use data to make decisions, 70-80% of the US truck market in owner operator and thus do not have the resources for making decisions based on data derived insights. The market conditions of freight change every hour in every zip code or market. There are 135 markets in USA and thousands of ZIP codes. Weather fluctuations are random and not accounted for in the planning. Planning a route which involves multiple cities and using multiple assets becomes a huge computational problem even for 20 cities. Using machine learning algorithms for designing routes which maximize profits could give smaller operators tools which would help them beat the market.
B) Load Cost Optimization: Usually a logistics company mixes the load volume between spot and contracts to have enough flexibility. And while contract rates are pretty much fixed at the beginning of the contract, spot rates are the loads that can either bring home the profits or be the pain point. Using machine learning algorithms to find out the optimized cost of a particular load based on various factors such as fuel cost, weather, stock market conditions, freight market conditions, government regulations and seasonal changes can help the dispatcher make an informed bid in the load boards and negotiate to profits.
C) Optimizing Document processing: There is a lot of documentation involved in the freight industry and most of it is physical paper. It is a cumbersome but necessary aspect of the business. Although the push is to be more paper free and going digital, you just cannot expect a sweeping change quickly. But Artificial Intelligence can help this through use of OCR, NLP, block-chain and other algorithms to quickly upload and process the documents saving up time and expediting the payment process. The average time of payment is assumed to be 30 days, that hurts the small players.
D) ELD Data: There was a mandate passed early 2018 to ensure that all long distance tractors and trailers be equipped with Electronic logging devices that stores data from the equipment. There are all sorts of data being stored in the device now ranging from HOS, speed, on/off-duty time, braking data, engine performance, driver activities etc. This much data can be useful in many ways, such as preventive maintenance of equipment, driver compliance, increasing asset utilization, tracking loads and implement higher service levels.
E) Single platform: This is not so much machine learning solution but a process improvement method that helps capture meaningful data that makes the data science life-cycle easier to implement. There are multiple platforms being used by a logistics team, load-boards, TMS, billing platforms, ELD dashboard, brokers, shippers etc. This makes decision making more difficult and requires incessant communication. Creating a single platform that uses data from all these platforms and presents the key action points and deliverable is a useful asset.
Useful Data resources:
Freightwaves is data aggregation company that provides Sonar which is a platform as a service giving out data in different granular levels, from tender rejection to produce volume, there are a lot of data streams that could be helpful for managers to make decisions.
DAT operates the largest truckload freight marketplace in North America. Transportation brokers, carriers, news organizations and industry analysts rely on DAT for market trends and data insights derived from 256 million freight matches and a database of 65 billion of market transactions.
TMS is often the most overlooked data source, the data is personal to the company and thus has huge value, using visualization tools to can help find out many hidden patterns.
RELATED POSTS
View all